2024 Bad Bot Report Explore the latest trends & impact of unmanaged bot traffic
Get the reportComprehensive digital security
Imperva latest news
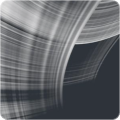
KuppingerCole Report: Why Your Organization Needs Data-Centric Security
Read more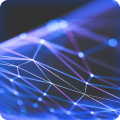
Data Security in 2024: Unveiling Strategies Against AI-Driven Cyber Threats
Watch webinar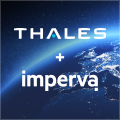
Thales & Imperva join forces to create a global leader in cybersecurity
Find out moreEnterprises move to Imperva for
world class security
Faster response
Accelerate containment with 3-second DDoS mitigation and same day blocking of zero-days.
Deeper protection
Secure applications and data deployed anywhere with positive security models.
Consolidated security
Consolidate security point products for detection, investigation, and management under one platform.
Imperva products are quite exceptional
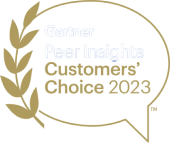